Author: Andrew Morgan, CFA
Much has been said about how artificial intelligence (AI) and machine learning might change the investment profession. Whether it is the advancement of automated trading, using alternative data to find new investment signals, or robo-advisors becoming more capable, the main focus to date has been on how these tools will cause the investment manager’s job to evolve, or even disappear under more extreme scenarios.
Some believe that not enough attention has been given to the second order impact of AI on the industry, and what I perceive to actually be the much larger and potentially more interesting outcome. By second order impact, I refer to the impact of AI on the industries and companies we analyse and invest in. Market fundamentals, financial analysis and sometimes just gut feel drives a lot of investment decisions outside of quantitative fund management, but how will this traditional analysis change in the coming decade?
While there are challenges around talent and finding high value problems to solve with machine learning, industries are well on their way to implementing models across their value chains.
Figure 1: McKinsey Survey on AI Adoption - November 2018
While some use cases are relatively benign and are mostly concerned with automating support functions, many use cases are becoming increasingly tied to core business models. Such an example is using more advanced modelling to help geologists in hydrocarbon exploration, an expensive and increasingly challenging activity done by large petroleum companies and governments.
If machine learning modelling becomes central to speeding up this process more accurately, very soon companies will be competing based on their data and modelling skills, and this will become crucial to their ongoing business growth. There are many such examples starting to develop across industries.
As an investor or analyst, how much do we know about a company’s AI activities? Annual reporting, past financial history and media releases are unlikely to help us understand who might be winning the AI arms race in a particular industry, unless perhaps they are a pure technology company.
While past impacts on company financials from technological innovations set some precedent, this is new uncharted territory. Analysing life insurance companies over the past few decades is potentially comparable –those companies identified with the better actuarial skills and models were the ones which tended to perform better in the long term, or at least were able to avoid catastrophic shocks in recessions and financial crises. However, the current landscape of AI and machine learning modelling in less regulated industries is still very much evolving.
It’s also not only about the performance and skill of data scientists in organisations that would be of interest in making investment recommendations. Much of the modelling done in information-based industries are currently skirting the lines of ethics and allowable use of personal information.
High profile issues are well known surrounding the likes of Facebook and Google collecting personal information and using it for their own gain. There are many smaller companies and start-ups who similarly do this, from personal health data to user behaviour, and their business models increasingly rely on being allowed to continue to do this.
All it might take is one data leak, a case of unintentionally disadvantaging a single demographic, or a change in regulation to cause these companies to have serious difficulties.
The challenge we have as an industry going forward, at least for those who will be working for the next 20-30 years, is understanding what we need to know about wider AI activities, and in how much detail, to make informed investment recommendations.
Regulators and financial reporting bodies will also need to decide on how to regulate AI, and what companies should publically disclose in order to help analysts and shareholders better understand the landscape and the risk.
The companies themselves should plan for a much more in depth AI assurance and validation process, which not only reduces their own risks of such experimentation, but allows them to better explain what they are doing in AI to their various stakeholders. Perhaps one day the ability to read a financial statement will be as important as being able to read and understand how a complex algorithm makes decisions.
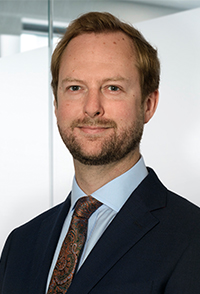
Andrew Morgan, CFA, is a Senior Manager at Deloitte working in the area of innovation and data science across financial services